Auto Theft in Vancouver:
Time, Transit, and Zoning
Discussion
Temporal Process
While Vancouver does not experience a high volume of car theft each month, the relatively consistent values allow for a more meaningful comparison. To enhance the usefulness of this data, arrests of auto thieves could be included in a further analysis to see if some of the drops in auto theft correlate with arrests. An example of where this could be useful is the month of February. Two potential options which may explain the low levels could be winter weather acting as a deterrent, or that a significant arrest, or even multiple arrests, was made either at the end of January or the beginning of February.
The dispersed nature of auto thefts in Vancouver by month stolen has interesting implications. This pattern may suggest that thieves are aware of the location that cars were stolen from and avoided those locations for some period of time. Because the relationship is not completely dispersed, however, it is more likely that individual thieves are not communicating between each other but dispersing their own thefts such that a random pattern is created.
The consistent central mean of the auto theft locations separated by month is very useful information. While some thefts occur in the location of the central mean, it does not have the highest density of thefts. Its location, however, does suggest that the majority of auto thefts occur towards the north east of the city, with other notable concentrations Downtown, in Kitsilano, and near the Oak Street Bridge.

Bus Stops and Auto Theft
While Vancouver has a comprehensive transit network that provides service to the majority of the city, the generation of a layer of random points, meant to simulate potential car thefts, within Vancouver shows that the actual location of auto thefts occurs much closer to bus stops than the random points. The much smaller mean distance from bus stops for the auto theft may be interpreted as the majority of auto theft points occurring within and near the bus network. The maximum distance of 514m, however, is just as important as it is dwarfed by a maximum distance of 17011m for the random points. Not only are the auto theft points on average closer to bus stops, but even the farthest values, of which there are few, are not very far away.
The average nearest neighbour summary for the auto theft layer produced a dispersed result. It was not extremely strong but was found to be significant. This is most likely due to the fact that the bus network itself is dispersed. When examining the spatial autocorrelattion report for the distance to the bus stop for the auto theft layer, however, a strong clustered result is generated. As 60% of the auto theft points had a distance to the nearest bus stop below the mean, the clustered spatial autocorreltaion report results acts as evidence that the majority of thefts in Vancouver are clustered close to bus stops.
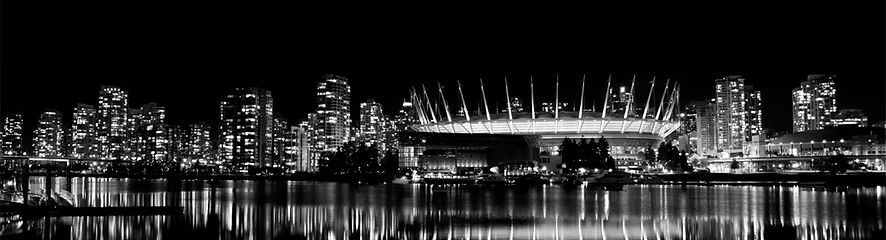
Zoning Process
The zoning variable incorporates an interesting component to the explanation of auto theft, as it attempts to explain why cars would be in that area. With this in mind, zones may either attract cars during the day, such as commercial, attract cars during the night, such as residential, or both like the comprehensive class. An important assumption made about zoning districts in this project is that zoning districts are relatively homogenous areas regardless of where they are in the city. With this in mind, the percentages of crimes per zoning type appear to explain the relationship. That conclusion, however, does not take into account the significant differences in area that each zoning district covers. By normalizing the data by total area of the zoning district, a more accurate depiction of the risk of theft within a particular zoning district is created. A particularly large source of error that is present in this method exists for the data points that are very close to boundaries between zoning types. In this situation it is not clear which potential zoning type acted as the attraction for theft. An even more complicated option that could potentially occur is that one zoning type attracts the criminal, while the another type provides the opportunity for theft. Using the commercial zoning district as an example, when a sixty meter buffer is applied to the class, the total number of encompassed thefts increases from 31 to 70. This dramatic shift adds a significant degree of error regarding the effect of zoning districts on auto theft. Below is an image representing this change. Just in the small frame located near Broadway and Kingsway, only three auto thefts occur within the commericial zone. When using the sixty meter buffer, however, the number of thefts increases by 100% to 6 thefts.
